We scooped an award for our Real-Time Networks project in the ‘Energy Data Collection and Analysis Project of the year’ category at this year’s Energy Awards, held at the London Hilton on Park Lane last month.
Since 2016, our Real-Time Networks project has been conducting the most extensive and meaningful gas demand research the industry has seen for decades. We’re developing the world’s first real-time gas demand network model, which is a critical step in our ambition to decarbonise the gas which flows through our network. The aim of the project is to demonstrate a flexible gas network can meet Great Britain’s current and future energy needs for a more efficient, low-carbon and affordable gas future.
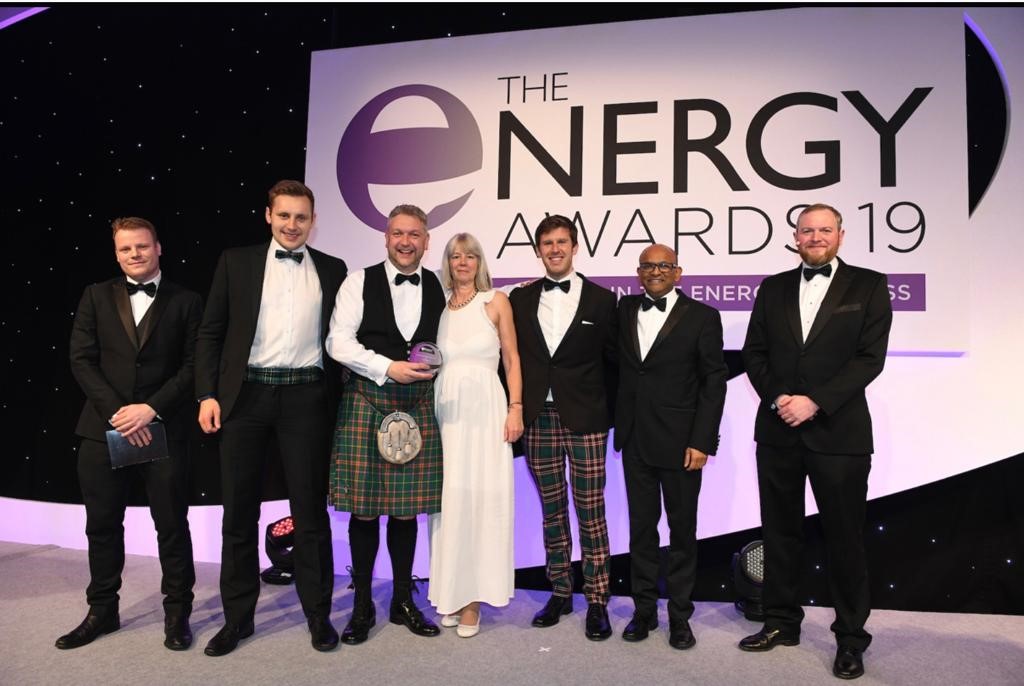
Our team accepting the award
Each year, the Energy Awards celebrates the best in our industry, recognising the intelligent use of energy. Our Energy Futures Project Manager Alexander Webb said: “We’re delighted to receive this award on behalf everyone at SGN involved our ground-breaking research project. It’s great to be recognised for the innovative work we’re carrying out, and this project represents a step change in the industry and is an essential part of ensuring the gas network plays a key role in a lower carbon energy future.”
Our trial is based in our south-east patch of the gas network, and novel use of technologies installed at key points along the network have enabled live monitoring of gas performance and consumption data every six minutes. Our steams of live data are analysed as it feeds into our real-time network model. Our project is due to complete by Summer 2020 when the full model will be developed, and the results can be published.
The Energy Awards 2019 judges said: “We appreciate the work being done in an important area of decarbonisation, where the data collection is expected to have a major effect on delivery of future low-carbon heating. Real-time modelling will lead to expected reduction in peak demand.”